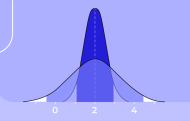
What we learned from running 200+ experiments on CUPED
Making experiment data-based decisions quickly and with confidence is a delicate art where experimenters have to find the balance between speed and accuracy. Peeking at experiments might provide insights faster; however, looking prematurely – before reaching statistical significance – jeopardizes accuracy. So experimenters need help to get to results faster safely.
Since January 2023, the Kameleoon team has been internally testing CUPED, one of the most powerful methodologies for accelerating experiment results, while increasing data accuracy.
After three months of testing, we concluded that applying CUPED to an experiment’s results can lead to a potential reduction in required sample size up to 60%. This means that CUPED, when applied to the right type of experiments, can empower our users to:
- Accelerate their experimentation and personalization velocity
- Make data-driven decisions faster and with more confidence
- Gain better estimates on the effect of experiment variations
- Reduce the risk of false positive results for tests with fewer data points (i.e., campaigns with smaller sample sizes)
What is CUPED and how does it yield more accurate results, faster?
CUPED (Controlled Experiment Using Pre-Experiment Data) is a powerful variance reduction technique where we use pre-experiment data to increase the precision of our estimates and reduce confidence intervals and pre-exposure bias in experiment results. CUPED was introduced by a research team at Microsoft in 2013 as a way to improve the sensitivity of online controlled experiments, and has been leveraged by top tech companies, like Facebook, Netflix, and Airbnb.
Applied to the right experimentation use cases (more on that later!), CUPED can significantly decrease:
- Confidence intervals
- p-values
- Required sample sizes
- The duration for which experiments need to run
Simply put, this technique provides you with faster test results, better effect estimations, and more confidence in your tests.
How did we test CUPED?
Based on the Microsoft team’s recommendations, we knew we wanted to reduce variance by looking for correlations for the same variable that was shared across previous experiments and a live experiment.
We computed the correlation between the conversion of the main goal of a live experiment and its conversion during the 2 weeks preceding the start of the experiment for over 200 experiments. Based on this correlation, we estimated the required increase in sample size to get a similar effect..
In the course of our tests, we checked whether visitors exposed to the current experiment:
- Engaged in a session in the past two weeks,
- Converted against the main goal of the current experiment during that two-week period, then
- Converted against the main goal of the current experiment while the current experiment was live.
We then computed how much the visitors' behaviors prior to the launch of the experiment correlated with their behaviors during the experiment.
The formula for CUPED tells us, the more visitors’ behaviors correlate, the more reduction in variance we will experience. Additionally, because there is a relationship between variance and sample size, we can compute the impact of CUPED by understanding how large of a sample we’d need to reduce the variance to the same extent that CUPED did.
When to apply CUPED?
While CUPED can be impactful, not every experiment needs or benefits from its application. In our tests, we saw that the effect of CUPED varied among users’ vertical, visitor population, and main goal.
And, as mentioned above, there are experimentation use cases where it makes more sense to apply the technique. We have found that CUPED will have the most impact in the following use cases and circumstances:
- Your experiment includes returning visitors: We can improve our predictions when the experiment is exposed to returning visitors because we’ll have data on them.
- You’ve already run many experiments in Kameleoon: The more you use Kameleoon, the more experiment data you’ll have that our algorithm can use to improve the effectiveness of CUPED
- There is a correlation between the goal conversions before the start of the experiment and during the live experiment: Make sure you’ve been collecting data for the main goal of your current experiment (for which you want to enable CUPED) prior to launching the experiment. The more correlation we have for the goal conversions of the previous and current experiments, the better we’ll be able to predict the real impact of the current test.
- To get the most out of CUPED, we recommend applying CUPED only to the key goals involved in your decision-making, like transactions.
How can Kameleoon users access CUPED?
You can enable CUPED on the Results page of individual campaigns. We don’t automatically apply the technique to experiments, as we believe you would know best when to turn on variance reduction, depending on the planned length, KPIs, and main purpose of your experiment.
However, note that once you enable CUPED, you need to commit to relying on its results. Switching between the original and CUPED-adjusted results and making decisions based on the more favorable outcomes will increase your probability of overestimating the lift of your experiment and risk of getting false positive results.
In the coming months, we’ll be monitoring the performance of CUPED and evaluating opportunities to further enhance this feature, for instance, by offering a custom lookback period to accommodate long-running tests, and more.
To learn more about how to apply CUPED to your results in Kameleoon, refer to our CUPED technical documentation.