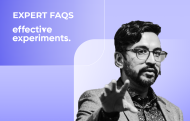
How can program management help you to scale experimentation across teams?
This interview is part of Kameleoon's Expert FAQs series, where we interview leaders in data-driven CX optimization and experimentation. Manuel Da Costa founded Effective Experiments 10 years ago to help organizations make better decisions through experimentation. He and his team have worked with some of the world’s most well-known brands to help them standardize, centralize, and scale testing capabilities.
What elements of program management need to be in place to scale experimentation across teams?
The foundational element of any experimentation program is “why.” “Why are we running an experimentation program?” and “What do we want to achieve?”
With the “why” answered, the most important foundational pillar is “Governance and Guardrails.” This pillar asks:
- “How do we ensure experimentation is executed and analyzed correctly?”
- “What checks and balances will ensure people don’t cut corners in the established processes and don’t cherry-pick the results?”
Governance dictates the rules, processes, and workflows needed and identifies who makes decisions in the experimentation program. With this in place, your experimentation program performance will improve, and you will gain support and trust across the business, helping you to scale across teams.
How can experimentation teams effectively communicate test learnings with the C-suite and broader business?
To communicate to the C-level, teams must realize one fundamental truth—stakeholders don’t care about experimentation like Conversion Rate Optimization (CRO) teams do.
While CRO folk spend hours debating p-values and statistical significance and hold fail parties to celebrate how many times they failed, stakeholders only care about one thing—”how do the insights help us make better decisions?”
In the Effective Experiments Experimentation Ops Framework, we created the concept of a “structured insight” because the experimentation teams that effectively communicate make insights:
- Accessible. The insight must be findable by anyone in the organization. You can achieve this using a classification and categorization system of tagging.
- Targeted. The insight must be personalized to the audience that will read it. You may have to summarize it a few times for different audiences.
- Clear and simple. Remove jargon and data-heavy language and use simple statements that are easy to understand.
- Actionable. An expert should be able to translate the insight into what action must be (or could be taken.) Actionable insights help stakeholders connect the dots easily without having to figure it out for themselves.
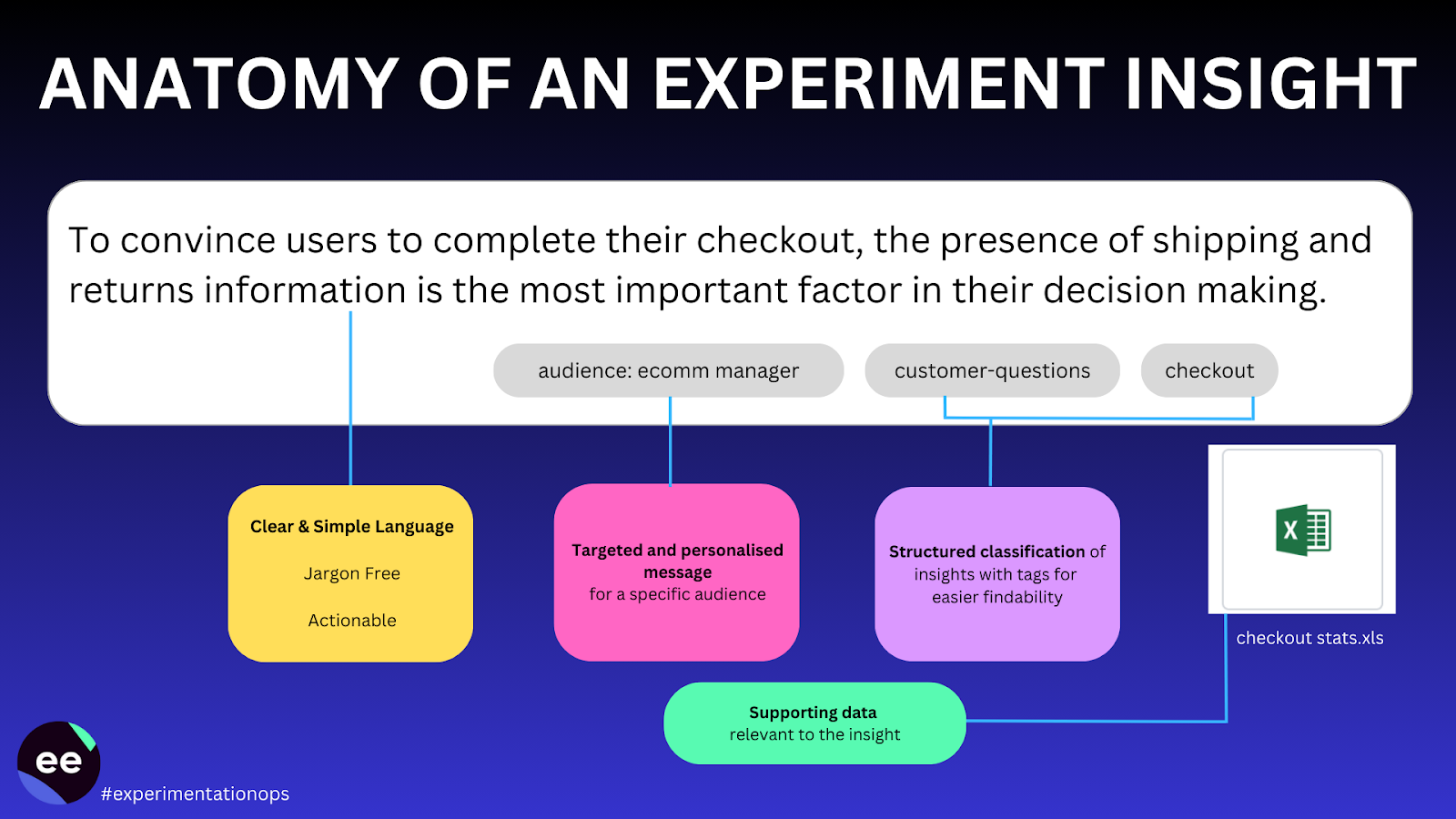
Example of a “structured insight”
What checks and balances should be built into program management workflows to ensure teams deploy quality experiments?
A solid governance framework will help create the checks and balances that are essential for ensuring the integrity of the decisions made from that data.
At a basic level, these are the checks and balances you should have in place:
- Process workflows. These should establish a transparent process for the whole experiment lifecycle. Create a RASCI model (Responsible, Accountable, Supporting, Consulted, and Informed) for each workflow step so it’s clear what needs to happen, who needs to action it, and who is responsible.
- A classification and categorization strategy alongside a system to tag each experiment. This must be reviewed regularly for erroneous use, spelling and classification errors, etc.
- Peer reviews, pre-mortem, and pre-test sessions. Establish regular team sessions to check whether an experiment has a sound foundation, clear hypothesis, and defined metrics.
- Post-test reviews. These are extremely important at the end of the process to understand how the experiment was planned, run, and analyzed. It is also a chance to ensure the validity and integrity of the data.
What metrics should teams use to assess the effectiveness of their experimentation program?
While most experimentation teams look at standard metrics like velocity, conversion rate uplift, etc., the Experimentation Ops Framework recommends teams look at the bigger picture using the following:
- Experiment health score: A way of tracking the validity of each experiment. This scorecard lets you understand if the experiment was conducted rigorously in the planning, live, and analysis phases with data integrity in mind.
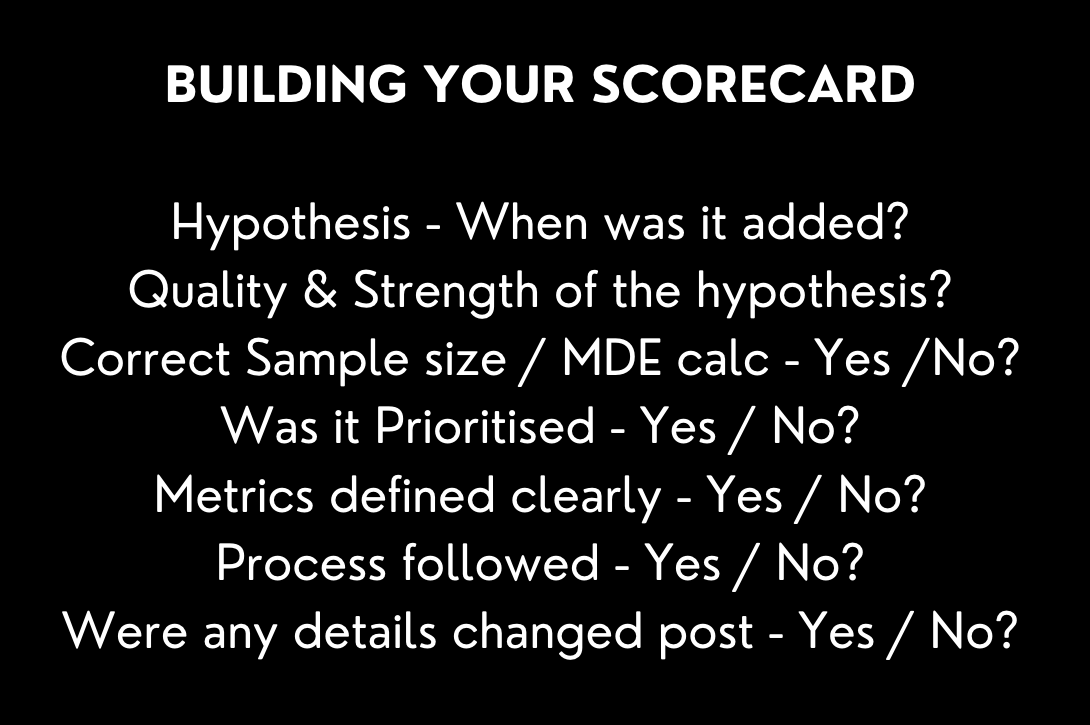
Experiment health scorecard
- Implementation lag time: How long winning experiments take to be implemented into production.
- Bottlenecks: Where are experiments getting stuck in the process, and for how long?
- Decisions made from insights: How many impactful insights resulted in action by stakeholders?
- Program growth: How much has the experimentation capability grown in the business?
- Ramp-up time: How long does it take someone new to adopt the processes and run their own experiments?
- Stakeholder engagement: How much engagement are you getting from stakeholders? How often are stakeholders commenting on the content you share with them?
How can experimentation teams align testing roadmaps with product and marketing teams?
Simply put, the alignment must start at the management level. Often, conflicting Key Performance Indicators (KPIs) and goals create unaligned teams, roadmaps, and plans. If you can get the senior management to understand the importance of coordination and orchestrating the roadmaps, you’ll be on to a winner.
Roadmap alignment is beyond the remit and ability of individual practitioners as they often need help to influence C-level decision-makers.
Manuel, you’ve been promoting lean methodologies since 2012. How has this influenced your experimentation work to date?
There are so many similarities between lean startup methodologies and experimentation. For example, they are both focused on testing assumptions and iteration.
Lean methodologies influenced how I set up Effective Experiments at a time when no one thought about experimentation program management. It helped shape the Experimentation Ops Framework, which came about by testing new approaches in program growth with our customers. It followed the build > test > learn cycles of the lean startup process.
Experimentation Ops is rooted in behavioral science and organizational dynamics. It is focused on people and facilitated by technology. The strategies started as suggestions to address challenges our customers faced, which they then implemented and reported on the outcomes. Consistent refining of these strategies led us to the Experimentation Ops Framework as it is today, but it's still evolving.
If you’d like to keep up to date with all the industry's top experimentation-related news, sign up for our newsletter or find industry leaders to follow in our Expert Directory.