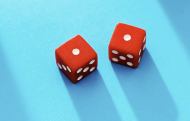
A/A Tests: How to use A/A testing in your experimentation program
To run an A/A test or not is the question that has different answers depending on which A/B testing expert you ask.
For some optimization experts, running an A/A test is out of the question. It takes too much time and pulls you away from running A/B tests that could bring in significant results.
Other experts disagree. They say A/A testing can be incredibly beneficial and is, in some cases, an absolute necessity.
In this article, you will learn:
- What A/A tests are
- How to run an A/A test
- The limitations of A/A tests
- The difference between A/A tests and A/B tests
Let’s dive in!
1What is an A/A test?
An A/A test is an experiment where you test two identical versions of an element. Traffic to the element is divided equally in two, with each group exposed to the same variation.
Unlike other forms of split testing, A/A tests are not focused on conversions. Rather making sure your results are valid and your systems are working as intended.
If the results of the A/A test are consistent, it means that any differences in the results of future A/B tests can be attributed to the changes made to the product, rather than something else.
2Why do companies run A/A tests?
There are various reasons why a company would want to run an A/A test.
1. Check for data accuracy
One of the number one use cases for running an A/A test is to check that the data being collected is accurate.
When you run an A/A test, you split traffic between two identical variations. Because these variations are exactly the same, you would expect the results of the test to not be statistically significant.
This then indicates that you are collecting accurate and reliable data.
Ronny Kohavi, Author, Technical Fellow, and A/B Testing and Experimentation Expert, believes A/A tests are hugely beneficial for ensuring data accuracy. He suggests running A/A tests online and offline to ensure you’re getting accurate data.
Lucia van den Brink, Senior Experimentation Strategist at Speero and Consultant at Increase Conversion Rate, also warns of the dangers of not doing A/A testing:
It can cause irreversible trust issues with the stakeholders or clients, and they might even want to turn away from experimentation. There are many things that can go wrong with a faulty technical or data setup, which you can catch early with A/A testing.
2. Identify Sample Ratio Mismatch
Sample Ratio Mismatch (SRM) is an experimental error where traffic is disproportionately allocated to each variant. It indicates that something went wrong with the experiment and the results are not valid.
For example, if a company intends to allocate 50% of its users to the treatment group and 50% to the control group, but due to technical issues or other reasons, 60% of users end up in the treatment group and 40% in the control group, there is a Sample Ratio Mismatch.
Running an A/A test can help you see if SRM is occurring in your experiments. Traffic during an A/A test should be split 50/50. If this is not the case, chances are, SRM is occurring and needs to be addressed.
3. Validate the configuration of an A/B testing tool
When you have chosen and implemented a new A/B testing tool, an A/A test is a good way to validate the configuration of your testing tool.
Shiva Manjunath, Experimentation Manager at Solo Brands, recommends running an A/A test as the first thing you do after setting up or switching to a new A/B testing tool.
By testing the same versions of one element with the same goals, you can establish if any changes in your results are coming from how you installed your A/B testing tool.
4. Establish a baseline for conversions and sample sizes
Another reason why you may choose to run an A/A test is to establish a baseline for conversions.
Let’s say you run an A/A test to identical versions of a landing page. Version A has a conversion rate of 3.51% and the second version A has a conversion rate of 3.52%.
You can now put your baseline conversion rate somewhere between 3.51-3.52%.
Going forward, you can keep this baseline metric in mind to determine a range of conversion rates that you expect to see from an A/B test, as well as the conversion rate you would like to exceed.
A/A tests can also help you determine the minimum sample size you need to run A/B tests. Say you run an A/A test that takes 3 weeks to reach statistical significance and you send 20,000 visitors to both A versions.
You can easily calculate the minimum sample size you will need for A/B tests from the size of your audience bucketed in the A/A test you ran.
3Limitations of A/A testing
The biggest reason why experimenters choose not to run A/A tests is because they are resource-intensive.
A/A testing takes time and resources to implement. In resource-scarce environments, this can be costly. When you are focused on delivering value and improvements that lift revenue, waiting for A/A tests to conclude can feel like a waste of resources you could be deploying elsewhere.
Craig Sullivan, Optimizer in Chief at Optimal Visit, says it best:
While the volume of tests you start is important, how many you finish every month and how many from those that you learn something useful from matters most.
Running A/A tests can eat into ‘real’ testing time.
The opportunity cost of running an A/A test when compared to an A/B test is the primary reason you will find opposition to the practice of A/A testing.
Unlike A/B tests that tell you which version is better and what improvements you can make to customer experience to increase revenue, A/A tests do not tell you anything significant to your bottom line.
When you are in the business of increasing revenue, anything that doesn’t outrightly contribute to this goal can be seen as a waste of time and resources.
However, consider this - the cost of running an experiment and obtaining inaccurate data can be far greater than waiting a few weeks for an A/A test to run.
4How should you interpret the results of an A/A test?
In most cases, the results of an A/A test should be almost, if not, identical. The difference between the two numbers should not be statistically significant.
If there is a significant difference detected in the results of your A/A test, then something has gone wrong.
That could mean that:
- Your A/B testing tool is not configured properly
- Sample Ratio Mismatch has occurred
- The test was not set up correctly
- You’re dealing with a false positive, or a reported increase in conversion even though one does not exist
With a confidence index of 95%, the chance of obtaining a false positive is 5%. This figure can be skewed if we look at the results before the end of the test, also known as “peeking.”
This is because the confidence threshold set for a test actually applies to its entirety. It is therefore bad practice to look at this indicator before the test is completed because it breaks the rules of statistics.
5Example of an A/A test
Let’s say we want to look at two different cities with the goal of identifying which city has the older population.
The statistical method would consist of establishing two representative and sufficiently sized samples (one per city) and then comparing their average age.
In the case of an A/A test, we would select two groups of individuals in the same city. The correct statistical methodology involves using the confidence index that we wish to obtain (95%) to create the size of the sample to test (let’s say 10,000 people).
If we complete the study using this statistically valid number of inhabitants, no difference will be detected between our groups in the A/A test.
Where results can go wrong
Taking the example above, if we repeatedly look at the results before the end of the study, the possibility of seeing a false positive increases.
We may also get incorrect results if the sample size is too small.
If we put 20 people in each group, there is a high probability that one of the two groups will have a higher average age than the other group (even though it is the same city), because the samples are too small. If we repeat the same test with 20 people in each group, it is very likely that we will once again find an age difference.
This example clearly shows that to obtain a valid result, we need a sufficient sample size. And if we look at the test results too soon, we risk obtaining invalid results.
6A/A testing vs. A/B testing: What’s the difference?
The difference between A/A testing and A/B testing is that A/A testing tests two identical variations of an element, while A/B testing tests two different variations of an element.
Measuring different variables
One key difference in A/A tests and A/B tests is found in the variables they seek to measure. In A/A tests, you compare the same element without any differences in any of the variables. It’s essentially comparing green apples to green apples.
In A/B testing, you compare two or more (in the case of A/B/n tests) versions of the same element with one variable changed in each variation. You take an original page and pick a variable to change, say the color of the “add to cart” button.
If your original page has a yellow “add to cart” button, you design different versions of the same page with the variable “add to cart button color” changed from yellow to black in version B, yellow to blue in version C, and so on.
The sample size required to come to a valid result for A/A tests then is significantly larger than what you need to run A/B tests.
Different end goals
Another key difference between A/A and A/B testing lies in their end goals.
In A/A testing, the end goal is to check the accuracy of your A/B testing tool implementation and the validity of your optimization process. The end goal of the A/A test doesn’t directly lead to conversions.
A/B testing, on the other hand, is focused on finding versions and variables that impact your primary metric, usually conversions. In A/B tests, you find out which version affects your bottom line and improves the experience for your customers.
7A/A/B tests: are they better than typical A/A tests?
A/A/B tests are gaining popularity because they solve one of the biggest problems that veteran optimizers have with A/A testing - the problem of A/A testing taking time away from A/B testing that can lead to significant wins for your website.
By running A/A/B tests, you are able to combine both an A/A test and an A/B test. Since the results of the A/A tests are independent of the results of the A/B test, A/A/B tests are a compromise between running both kinds of tests separately.
The results of your A/A variants can validate the results you get for the B variant. If your A variants do not produce any alteration in your conversion rates, it can give you the assurance that the results you are getting for variant B are valid.
In short, running A/A/B tests helps you validate your A/B testing tool configuration (a big advantage of running A/A tests) while also optimizing processes, elements, and variables that can lead to notable improvements to your website or product.
A caveat with running this type of test is that the A/A variants will still take time to run. This is due to the sample size being smaller for both variants. So, your A/A/B tests will likely still end up taking more time to run than a typical A/B test.
8How to run an A/A test
Running an A/A test is relatively straight-forward. Below are five steps you can follow to run an A/A test in your A/B testing tool.
- Set your goals: Before you start, determine what the goals are for your A/A test. Determine what you want to test and what you want to learn by establishing a clear hypothesis, metrics, and KPIs to measure.
- Divide users into two identical groups: Create two identical groups of users. Each group should be more or less the same size and have similar demographics and characteristics.
- Show the two groups the same version of an element: Set up your tests so that the two groups are both shown the same version of your website, app, or product for the same amount of time. Because you want things to be as equal as possible, make sure that the user experience is consistent across both groups.
- Analyze the results: Once your test is complete, analyze the results and interpret what they mean. If the results are consistent between the two groups, then you can conclude that your testing tool is configured properly and any future test results can be trusted.
If the results are not consistent or if a difference is detected where there is not one, investigate the cause of the discrepancy to determine your next steps.
Conclusion
Although some experimenters make a case against A/A testing, most experts agree they are an essential part of a mature testing program.
They help establish a baseline of conversions, validate the implementation of your A/B testing tool and confirm that the data you’re collecting is indeed correct.
While A/A tests require time, resources, and a large sample size, it is still valuable to use them when the need arises.
To learn more about the importance of data accuracy and how Kameleoon can help you obtain accurate data, visit our data accuracy specialty page.