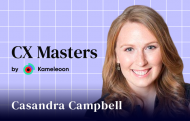
How top e-commerce stores boost their A/B test success rate
Millions of shops run on Shopify but only a few master the basics of creating data-based hypotheses, testing and iterating.
Over the past seven years, Casandra has gathered insights from across a broad range of different e-commerce sectors and different-sized businesses, from small mom-and-pop storefronts to large national brands.
She’s seen too many e-commerce stores dive straight into more complex optimization tasks thinking that’s where the real growth lies. And then they’re shocked when results don’t immediately follow. She knows better: solid growth comes from solid fundamentals, including making hypotheses based on observed customer behavior.
If you want to optimize your conversions, you first need to optimize your testing
It is clear to her that there is no ‘magic bullet’ test to eliminate cart abandonment or increase every order value. Instead, the secret to optimizing a successful e-commerce shop is to apply the fundamentals again, and again, and again.
See more CX Masters videos
Stop guessing and start using hypotheses
Casandra believes too many experimentation teams mistake guesses for hypotheses. They also often over-rely on expert or executive opinion—something critics of this thinking nickname “highest-paid person’s opinion” (HIPPO).
Instead of suggesting actual tests, these teams just propose changes based on those opinions. Anyone who has built an online shop probably has experienced a situation where an executive swoops in and says, “I really think these images could be cooler. Let’s use younger models.” Or, “Let’s change the button color. Pink will work better.” Those are guesses about conversions, not hypotheses.
A hypothesis is more than just an educated guess
A hypothesis is a proposed explanation for a customer's behavior. That explanation must be informed, and crucially, it must be testable.
You should be able to form a valid hypothesis into an if-then statement. For example, “Data shows that our Shopify store customers prefer warmer colors. We hypothesize that if we change our purchase button to a warmer color, like pink, then we’ll see an increase in order sizes.” That is a testable assertion.
Get your 3-step fundamental testing process perfect
You don’t need—or even want—to set up every test to find a big revelation. Instead, Casandra suggests you pick something smaller for your next test and focus on perfecting the 3-step fundamental testing process.
Step 1: Craft a data-based hypothesis
To form a hypothesis, you first need some kind of data. That data might come from analyzing your funnel, user surveys, or researching what’s worked for other companies through websites like Baymard or GoodUI.
Then decide what informed question you can ask based on the insights collected from that data. For example, maybe you’re struggling with cart abandonment. So you look at survey responses, and one positive you consistently see from customers is that they like that your products are sustainable. Great! That’s an insight we can latch onto.
So your hypothesis could be, “Data shows our customers like sustainable products. If we change the copy on all product pages to emphasize sustainability, then we hypothesize that order size will increase.” That is a well-crafted, testable hypothesis.
Step 2: Conduct a test to challenge your hypothesis
The next step isn’t just to make a change. You need to conduct a controlled test. One of the best and most straightforward is still an A/B test. They are among the most reliable methods for getting clear, actionable data about customer behavior without any guesswork.
However, in order for your test to be trustworthy, you need to do some things ahead of time to make sure you have a good experimental design. For example, you need to be sure to collect enough data to detect an effect size that’s meaningful for your business.
In other words, you need to determine whether your test will run for 2 weeks or 2 months to drive a big enough impact to your business. Do you need a PPC budget or several thousand dollars or perhaps several tens of thousands to get enough traffic for a scientific test? This depends on how much uplift you will need to justify the test from a business perspective.
Let’s return to our increased order example. Our e-commerce company sets up an A/B test where language around sustainability is added to B variant product pages served to exactly half of all sessions. You can use an A/B test calculator to determine the length of the test.
Step 3: Analyze and iterate
Once you’ve finished collecting data, it is time to analyze the results. In our example, the test revealed something curious: order size increased on B variant pages, but only slightly, and only when customers bought consumable products. Carts with durable goods were unchanged.
Then take your test results and use them to inform your next test. That is iteration. In this case, the next hypothesis would be that, in the case of consumable goods, customers prefer sustainability. However, perhaps they believe durable goods don’t have as much environmental footprint and therefore sustainability is not a relevant UVP.
You’re building on previous quantifiable results to obtain new and better results in the next test. In other words, you will have a powerful feedback loop using the 3-step testing process.
A reliable result is always a good result
Casandra Campbell points out that you’re not trying to find a magic bullet. Instead, you’re trying to build a reliable process for steadily improving conversions. If your process is sound, then the results will always be good.
All results are good because they provide an insight about your customers that you can trust. In our example, our experimentation team learned their customers care about sustainability, but it really only affects their purchasing habits of consumable products. That’s a meaningful insight!
Look to build a testing process on a solid foundation. Do that, and you can trust the results that follow. Each insight will lead to another hypothesis you want to explore.
Want to get ahead of your competition and create experiences your customers love?
Click here to learn how to prioritize and build a testing roadmap.