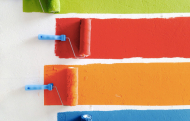
What is Multivariate Testing? Benefits & Examples
ACADEMY / A/B testing training blog
Multivariate testing (MVT) is an A/B testing methodology that lets you test multiple variations of an element (or more than one element) on your website at the same time during an experiment.
Multivariate testing (MVT) is a form of split testing. While it can be a very effective way to run a test on your website or product, you need to follow best practices to get significant, reliable, and actionable results.
In this article, you will learn:
- What a multivariate test is
- How it differs from A/B and multipage testing
- Limitations and benefits for its use
- When to deploy MVTs
Let’s dive in!
1 What is multivariate testing?
Multivariate testing (MVT) is a form of A/B testing that involves testing multiple variables, in different combinations, at the same time.
The idea is to simultaneously test all of the possible combinations of multiple variables that are running on the same page. From here, you can see which of these combinations has the biggest effect on your conversion rate or any other metric you’re measuring.
A good example of MVT is testing different combinations of copy and color for a specific call-to-action on a webpage. Instead of testing each variation against the control one at a time like in a split test, you can test multiple variations against a control all at once.
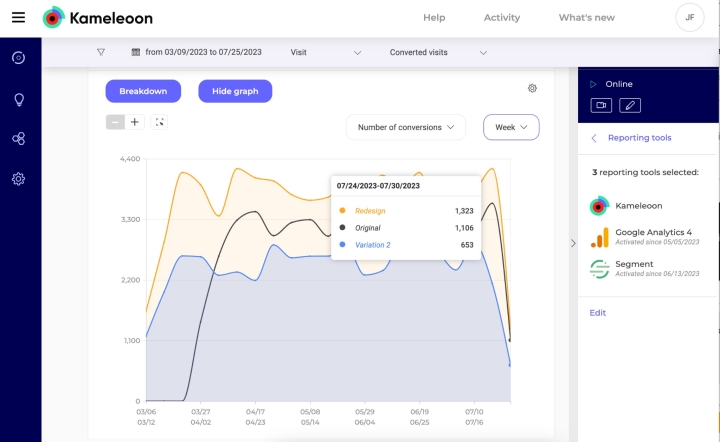
2 Benefits of multivariate testing
There’s a few reasons why many advanced experimentation leaders consider multivariate testing a staple in their testing program.
1. Helps identify the specific elements that influence conversion
Multivariate testing allows for a greater understanding of the reasons behind performance differences between different variations compared to a classic A/B test.
By systematically breaking down potential changes into combinations, you can isolate the key elements on the page being tested and measure the influence of each of them.
Jake Sapirstein, Head of Strategy at LiftCentro, explains.
A multivariate test lets you better understand how different elements interact with each other and which ones are instrumental to improving performance.
2. See results faster
Automatically creating and testing all potential combinations of a specific change can help you see results faster.
Rather than testing each variable against a control one by one, you can test multiple variations at a time and therefore see results faster than individual testing.
Daniel J. DeSimone, Conversion Rate Optimization Manager at PayScale, explains:
3. Increases your chance of a significant win
One benefit of running multivariate tests is that your chances of significant wins increases.
Because you’re testing so many combinations, the chances of you hitting a winning combination is a lot higher than if you were to run a series of tests on their own.
Shiva Manjunath, Experimentation Manager at Solo Brands, puts it this way:
4. Uncover interaction effects
An interaction effect is defined in statistics as “the role of a variable in an estimated model, and its effect on the dependent variable.”
Understanding interaction effects is important in understanding what variable or variant is responsible for the change that is occurring.
By nature, multivariate testing can help you find out the effect that different elements on your page have on each other - and how these interactions then impact your conversion rate.
3 Limitations of multivariate testing
There are two primary limitations of multivariate testing.
1. Requires a larger sample size
The more variables you add to a multivariate test, the larger of a sample size you need. Because you’re sending traffic to six or more variations in some cases, the sample sizes can get very large, very quickly.
Generally speaking, you’ll obtain an acceptable reliability rate in an A/B test in web experimentation from:
- 1,000 visits per variation if the page being tested has a high conversion rate (such as engagement on a landing page or click-through rate for a CTA button), and where the expected potential gain is high (for example, +10% or 20%).
- 7,500 visits/variations if the conversion rate is low (<5%), for example in forms.
Reaching 7,500 visits per variation takes time and can be complicated. This makes it very difficult for companies without hundreds of thousands of unique monthly visitors to run MVTs.
While the numbers may be different, the principle holds true for feature experimentation as well.
2. Takes longer to run a test
Another limitation is it takes more time to run multivariate tests than simple A/B tests.
From the upfront cost of investing time into creating the different combinations to running the test itself until it reaches statistical significance, it can take a long time to run MVTs.
Jake Sapirstein, Head of Strategy at LiftCentro, agrees:
Although you may see more significant results, multivariate tests generally involve a lot more work to get up and running, which can be a blocker for some resource-strapped teams.
4 What is an example of multivariate testing?
Below we’ll explain a basic example of a multivariate test.
Observation:
You see that the call-to-action (CTA) on the homepage of your desktop application is not converting as well as you would like.
You’re not sure if it is the color blue, the word “Start”, or some combination of the two causing the issue. You want to see how the CTA button color and the CTA copy interact, so you decide to make changes to find the best combination that will increase clicks.
Experiment Design:
For colors, you decide to change the CTA button colors to red and yellow.
You also want to change the wording of the CTA, so you use “Buy” instead of “Start”.
When building out this test, you will have your original CTA wording and button color:
- Start + Blue button
You’ll also have five new combinations in your multivariate test, along with your control:
- Start + Yellow button
- Start + Red button
- Buy + Yellow button
- Buy + Red button
- Buy + Blue button
It should look like this:
After you design your MVT, you will end up with six different variations in total where you are testing two main variables - CTA wording and CTA button color.
Results:
After a few weeks, you notice that the red button with the word, “Start” performs best. You consider adding more element changes to continue to see how different versions perform.
It’s important to consider that the more elements you introduce, the more variations you will have. If you introduce a third CTA wording, “Get”, your combinations will rise from six to nine. Another color change will introduce another three variables, so on and so forth.
This is what makes MVT so complex, but very effective at giving you significant wins.
5 When should you use multivariate testing?
There are several scenarios where you may choose to employ multivariate testing instead of the traditional two-variable split test.
When there isn’t a clear hypothesis to be tested
The first stage of designing your A/B test is to generate a hypothesis based on observations, and map out the different changes you want to test.
However, sometimes it can be hard to pinpoint the individual element that will make the key difference. If your CTA is not performing as expected, is the problem the wording, the color, or the shape? It’s not easy to be sure enough to create a strong hypothesis right from the start.
In this case, a multivariate test can be helpful. It allows you to test several elements at the same time, and let your testing platform generate all the possible combinations for you. This means you don’t rule out any possibilities.
When you’re testing user flows
When you are trying to optimize UX flows, multivariate testing can be indispensable in this regard.
Jake Sapirstein of LiftCentro says, “I like to focus multivariate testing more on user flows that are self-contained from a UX perspective, or where users tend to travel through isolated time sequences. Examples include landing pages, eCommerce checkout flows and application or quote funnels.”
He continues, “these are all contexts that tend to be somewhat sealed off from the core website, where branding and design consistency are usually guarded with more vigor.”
When you need to test design elements and how that affects your users, multivariate testing is your best bet in making fast and impactful changes.
5 A/B testing vs. multivariate testing: What’s the difference?
The key difference between A/B testing vs multivariate testing lies in the number of variables and variations being tested at a given time.
In A/B testing, you pick one variable to test across many variations. For example, you can decide to change your “Add to cart” button to “Buy now”. You design a variation with the “Buy now” button and test it against the original.
In multivariate testing, you pick multiple variables to test in combinations that yield multiple variations. For example, you can change the wording on your cart button from “Add to cart” to “Buy now” and then change the color of your cart button from red to blue to end up with six variations, rather than two.
But what if the A/B test has three or more variations. Does that make it a multivariate test?
The short answer is no.
Shiva Manjunath, Experimentation Manager at Solo Brands, explains:
A/B/n tests that test one variable across multiple variations are not multivariate tests. For example, you can test your “Add to cart” button against two variations with “Buy Now” and “Add to Basket” buttons.
This test whilst having multiple variations is not a multivariate test as it is only testing one variable: the add to cart button.
6 Multi-page testing vs Multivariate testing: What’s the difference?
Multipage testing focuses on optimizing your customer’s journey across a series of pages while multivariate testing focuses on finding the best combination of variables to enhance your customer’s experience on your website.
Daniel J. DeSimone, Conversion Rate Optimization Manager at PayScale explains:
Both multipage and multivariate testing are resource intensive - they take time to build out and require a large sample size.
However, both can be incredibly valuable when it comes to determining the right combination, be it variables or pages, that lead customers to convert.
7 Server-side multivariate testing
Much of the conversation around multivariate testing is about client-side testing - like changes to button colors, copy, and design elements.
However, multivariate testing can also be useful in server-side testing or testing on back-end infrastructure. With server-side testing, you can test complex algorithmic changes or test on a mobile application.
With server-side testing, there is no flicker effect and the performance of your website is not affected in any way, making it a great option when running complex, multivariate tests.
Here is a helpful checklist breaking down what you need to switch from client-side to server-side testing if you’re interested in making the switch.
8 How to run a multivariate test
Running a multivariate test may vary depending on what A/B testing tool you use. However, here is a basic outline as to how to run a MVT.
1. Create your variations
As with a classic A/B test, the first stage is to create the variations you want to test.
Let’s return to our example in which you want to change both the background color and wording of a CTA.
In your A/B testing platform, such as Kameleoon, you create the sections of your test; here these are “Color” and “Wording”.
Then, for each section, you build the variations you want. For example, in the “Color” section, you’d create a “Yellow” variation, a “Blue” variation and a “Red” variation. In the “Wording” section, a “Start” variation and a “Buy” variation.
The platform will then automatically generate all the possible combinations of your different section variations.
2. Allocate traffic for your multivariable test
Now you have to decide how to allocate website traffic to the different combinations for the duration of the test.
There are two approaches for allocating your traffic: by section or by combination.
Traffic allocation by section
In our example, we have the Color and Wording sections.
- The Color variations are “Yellow”, “Blue” and “Red”
- The Wording variations are “Buy” and “Start”
By default, the traffic will be distributed equally among all these six variations. Allocation by section is considered the simplest method since you have fewer sections than combinations. Here, you will have to distribute 100% of your traffic between “Yellow”, “Blue”, and “Red”, and 100% of your traffic between “Buy” and “Start”.
The A/B testing platform will then automatically allocate traffic to each of the combinations.
Traffic allocation by combination
In our example, six combinations are possible:
- Start + Yellow button
- Start + Red button
- Buy + Yellow button
- Buy + Red button
- Buy + Blue button
You can therefore decide manually how to allocate your traffic to each of the combinations, or, simply split it equally between all of your variations.
This traffic allocation method can become difficult to manage if you have many different variations since the number of combinations increases exponentially.
However, it is useful in certain specific situations, particularly when you want to exclude certain combinations from your test.
Here’s an example:
You are testing changing the background color and the text color in a CTA. You don’t want your visitors to see the combination of black background/black text (because they would see nothing).
If you are setting traffic allocation by combination, you could manually allocate zero traffic to go to the black background/black text combination.
3. Set up the KPIs to monitor
Once you have allocated traffic to different variations, you can create the goals to be measured during the test.
Again, this follows the same approach as for a classic test. You will find all available information on setting up your reporting tools and your goals here.
4. Simulate the multivariate test before launching it
Before launching your test, you’ll want to make sure everything is running as it should. One way to do this is by previewing the test in simulation mode, which allows you to preview your multivariate test before pushing it live.
You can check that you are happy with your choices and they work seamlessly before putting the test live online.
5. Monitor the results
The final, and perhaps most important step, is to monitor your results and analyze, in detail, the level of impact each element has on your main KPIs.
From here, you can ensure that you are making the best informed data-driven decisions when it comes to changing your page.
Multivariate testing has many use cases in your optimization program. While it is labor and resource intensive, the results you get from running MVTs can be considerable especially if the primary metric you’re trying to optimize is conversions.
Looking for an A/B testing tool that can handle multivariate and complex testing? Check out our A/B Testing Checklist that covers essential criteria to look for in an A/B testing tool.